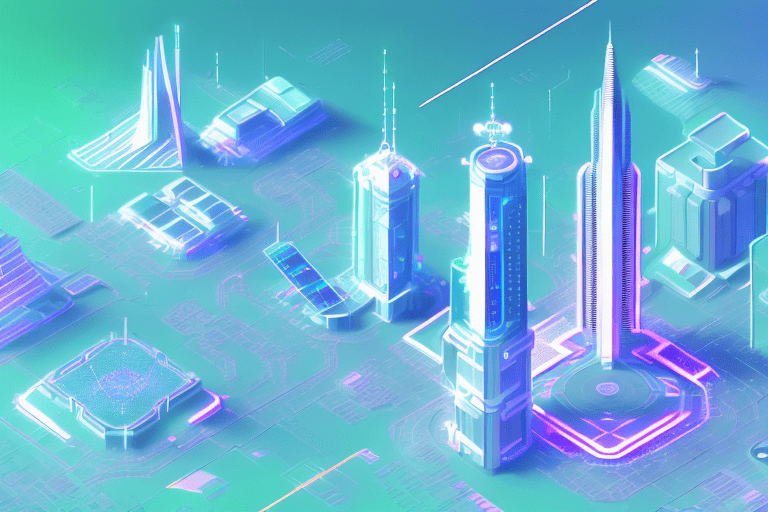
AI Risk Management: Strategies for Mitigating Risk in the Digital Age
AI Risk Management: Strategies for Mitigating Risk in the Digital Age
The adoption of Artificial Intelligence (AI) is rapidly increasing in various industries, including finance, healthcare, and transportation. This technological leap, however, comes with risks that require effective management to minimize harm to individuals, organizations, and society at large. In this article, we will explore the various risks of AI and provide strategies for mitigating them.
Understanding AI Risk Management
Defining AI risk management involves the application of processes and techniques to identify, evaluate, and prioritize potential risks associated with the implementation of AI. It involves identifying the risks that AI systems pose to businesses, users, and other stakeholders, and developing strategies to mitigate them.
AI risk management is a critical aspect of the development and deployment of AI systems. As AI systems become more advanced and are integrated into various aspects of our lives, it is important to ensure that they are safe, secure, and ethical. AI risk management helps to identify potential risks and develop strategies to mitigate them, ensuring that the benefits of AI are realized while minimizing potential harms.
Defining AI Risk Management
AI risk management is the process of identifying and managing potential risks associated with the development and deployment of AI systems. The process involves assessing the risks, developing a strategy for managing them, and monitoring and updating the strategy on an ongoing basis. The goal of AI risk management is to ensure that the benefits of AI are realized while minimizing potential harms.
Effective AI risk management requires a comprehensive understanding of the potential risks associated with AI systems. These risks can include data security and privacy concerns, ethical concerns around bias and discrimination, and the potential for AI systems to malfunction or make erroneous decisions.
One of the key challenges in AI risk management is the rapidly evolving nature of AI technology. As AI systems become more advanced and are integrated into more aspects of our lives, new risks may emerge. Effective risk management requires ongoing monitoring and evaluation of AI systems to identify new risks as they arise.
Importance of AI Risk Management in the Digital Age
The importance of AI risk management cannot be overstated. The digital age is characterized by the rapid development of technology, which has led to the widespread adoption of AI. This has created new opportunities for businesses, governments, and individuals, but it has also introduced new risks.
One of the key risks associated with AI is data security and privacy. As AI systems become more advanced and are integrated into more aspects of our lives, they may collect and process large amounts of personal data. This data must be protected to ensure that it is not misused or accessed by unauthorized individuals.
Another key risk associated with AI is ethical concerns around bias and discrimination. AI systems may be trained on biased data, which can lead to discriminatory outcomes. Effective AI risk management requires a comprehensive understanding of these ethical concerns and strategies for mitigating them.
Finally, effective AI risk management requires a commitment to ongoing monitoring and evaluation of AI systems. As AI systems become more advanced and are integrated into more aspects of our lives, new risks may emerge. Ongoing monitoring and evaluation can help to identify these risks and develop strategies for mitigating them.
In conclusion, AI risk management is a critical aspect of the development and deployment of AI systems. It involves identifying and managing potential risks associated with AI systems to ensure that the benefits of AI are realized while minimizing potential harms. Effective AI risk management requires a comprehensive understanding of the potential risks associated with AI systems, a commitment to ongoing monitoring and evaluation, and strategies for mitigating these risks.
Identifying Common AI Risks
As AI technology continues to advance, it is crucial to understand the risks associated with its development and implementation. By identifying these risks, we can develop effective risk management strategies to mitigate them. While the specific risks may vary depending on the context and application of AI, some common AI risks include the following:
Data Privacy and Security
Data privacy and security risks are a significant concern when it comes to AI. AI algorithms rely on large quantities of data to learn and make predictions. If this data is compromised, it can lead to severe consequences, including loss of trust, legal liabilities, and reputational damage. For example, suppose an AI system is used to process sensitive medical data. In that case, a data breach could result in the exposure of personal information, leading to significant privacy concerns and potential legal action.
Organizations must take steps to ensure that the data used by AI systems is secure and that appropriate measures are in place to protect against data breaches. This includes implementing robust security protocols, such as encryption and access controls, and regularly monitoring and auditing data usage to identify potential vulnerabilities.
Bias and Discrimination
AI systems are designed to learn from data, and if this data is biased, the resulting AI system will reflect that bias. This can lead to unfair treatment and discrimination against certain groups and individuals. Addressing bias in AI systems is crucial in ensuring that they are fair and equitable.
One example of bias in AI is facial recognition technology, which has been shown to have higher error rates when identifying individuals with darker skin tones. This bias can lead to misidentification and wrongful accusations, highlighting the importance of addressing bias in AI systems.
To address bias in AI systems, organizations must take steps to ensure that the data used to train these systems is diverse and representative of the population. Additionally, AI systems must be regularly tested and audited to identify and address any potential bias.
Lack of Explainability
AI systems are often described as ‘black boxes’, meaning that it is challenging to understand how they arrive at their decisions. This lack of transparency can lead to mistrust and skepticism from users and stakeholders, which can harm the adoption and potential benefits of AI.
For example, suppose an AI system is used to make lending decisions. In that case, it is essential to understand how the system arrived at its decision to ensure that it is fair and equitable. Without transparency, it is challenging to identify and address any potential biases or errors in the system.
To address this risk, organizations must prioritize transparency in their AI systems. This includes providing explanations of how the system arrived at its decisions and making the decision-making process as clear and understandable as possible.
Unintended Consequences
Another risk associated with AI is the potential for unintended consequences. For instance, an AI system designed to optimize traffic flow may lead to unintended outcomes such as increased congestion or environmental pollution.
Organizations must carefully consider the potential unintended consequences of their AI systems and take steps to mitigate them. This includes conducting thorough risk assessments and testing to identify any potential issues before the system is deployed.
Additionally, organizations must be prepared to respond to any unintended consequences that do arise. This includes having contingency plans in place and regularly monitoring the system to identify and address any issues that may arise.
Identifying and addressing the risks associated with AI is crucial in ensuring that these systems are safe, fair, and effective. By prioritizing data privacy and security, addressing bias and discrimination, promoting transparency, and mitigating unintended consequences, organizations can develop and implement AI systems that are beneficial to society as a whole.
AI Risk Assessment Framework
Artificial Intelligence (AI) is transforming the way organizations operate and make decisions. However, with the benefits of AI come significant risks that organizations need to manage. To effectively manage AI risks, organizations need a structured approach to identify and prioritize risks. The following framework can guide organizations in assessing AI risks:
Identifying AI Assets and Dependencies
The first step in the risk assessment framework involves identifying the AI systems and assets that the organization has in place. This includes not only the AI systems that are currently deployed but also those that are in development. It is essential to understand the dependencies of the AI systems on other systems, both internal and external to the organization. This includes understanding the data sources used by the AI systems, the hardware and software infrastructure, and the personnel involved in the development and maintenance of the AI systems.
Identifying AI assets and dependencies is critical to understanding the scope of AI risks and developing an effective risk management strategy.
Evaluating AI Vulnerabilities
The next step is to identify vulnerabilities in the AI systems. This involves assessing the AI system’s data architecture, model development process, and system infrastructure. It is essential to identify potential vulnerabilities in the AI systems that could be exploited by threat actors, including cybercriminals, malicious insiders, and state-sponsored actors.
Assessing AI vulnerabilities requires a multi-disciplinary approach that involves experts in data science, cybersecurity, and system engineering. This approach helps organizations identify potential vulnerabilities in the AI systems and develop effective mitigation strategies.
Assessing Potential Impact
In this step, the organization evaluates the potential impact of risks. This includes assessing the extent to which the risks may impact stakeholders and the organization’s reputation. For example, a data breach involving AI systems could result in the loss of sensitive data, damage to the organization’s reputation, and legal liabilities.
Assessing the potential impact of AI risks is critical to developing effective risk management strategies. This step helps organizations prioritize risks and allocate resources to manage the most significant risks.
Prioritizing AI Risks
Finally, risks should be prioritized based on their likelihood and impact. This involves developing a risk matrix that ranks risks based on their likelihood and impact. The risk matrix helps organizations focus their resources on managing the most significant risks.
Effective risk management requires a continuous process of identifying, evaluating, and prioritizing risks. Organizations need to develop a risk management culture that involves all stakeholders, including employees, customers, and partners. By following a structured approach to AI risk management, organizations can effectively manage AI risks and realize the benefits of AI.
Strategies for Mitigating AI Risks
As AI continues to advance, so do the risks associated with its implementation. Organizations need to be proactive in identifying and mitigating these risks to ensure that AI is used in a safe and responsible manner. Some strategies that can be implemented to mitigate these risks include:
Implementing Robust Data Governance
One of the biggest risks associated with AI is the potential for data breaches. To mitigate this risk, organizations need to implement a robust data governance framework. This framework should include data access controls, data quality checks, and regular audits of data usage. By implementing these measures, organizations can reduce the likelihood of a data breach occurring.
Additionally, organizations should consider implementing data anonymization techniques to further protect sensitive information. This can include techniques such as differential privacy, which adds noise to data to protect individual privacy while still allowing for accurate analysis.
Ensuring AI Transparency and Explainability
Another risk associated with AI is the lack of transparency and explainability in AI decision-making processes. This can lead to mistrust and skepticism from stakeholders, as well as potential legal and ethical issues.
To mitigate this risk, organizations should ensure that their AI systems are transparent and explainable. This can include implementing transparency mechanisms such as audit trails and documentation of decision-making processes. Additionally, organizations should consider using interpretable models, which are easier to understand and explain than more complex models such as neural networks.
Developing Ethical AI Guidelines
AI systems can also introduce risks associated with bias and discrimination. To mitigate these risks, organizations should develop and implement ethical AI guidelines. These guidelines should ensure that AI systems are designed and used in a fair and equitable manner.
Organizations should also consider implementing diversity and inclusion initiatives to ensure that the development and use of AI is representative of all individuals and communities. This can include ensuring that diverse perspectives are included in the development process, and that AI systems are tested for bias and discrimination.
Regularly Monitoring and Updating AI Systems
Finally, organizations should regularly monitor and update their AI systems to mitigate risks associated with unintended consequences. This includes ensuring that AI systems are continuously evaluated for potential risks and that updates are made as necessary to mitigate these risks.
Organizations should also consider implementing a feedback loop, which allows for continuous improvement of AI systems based on user feedback and real-world performance. This can help to ensure that AI systems are effective and safe for all users.
By implementing these strategies, organizations can mitigate the risks associated with AI and ensure that this technology is used in a safe and responsible manner.
Building a Culture of AI Risk Management
Building a culture of AI risk management involves ensuring that all stakeholders are aware of the risks associated with AI and are committed to mitigating them. This is especially important as AI is becoming more prevalent in various industries.
Artificial intelligence (AI) is a rapidly evolving technology that is transforming the way we live and work. AI systems are being used to automate a wide range of tasks, from customer service to medical diagnosis. However, with this increased use of AI comes increased risk.
Here are some additional ways to build a culture of AI risk management:
Training and Education for Employees
Organizations should provide training and education for employees on AI risk management. This will help to ensure that employees are aware of the risks and are equipped to contribute to risk management efforts. Training can include understanding the limitations of AI, identifying potential biases, and understanding how to interpret AI-generated results.
AI is only as good as the data it is fed, and if the data is biased or incomplete, the AI system will produce biased or incomplete results. Therefore, employees need to understand the importance of data quality and integrity when working with AI systems.
Encouraging Cross-Functional Collaboration
Effective AI risk management requires collaboration across functions and departments, including IT, legal, ethics, and compliance. Encouraging cross-functional collaboration can help ensure that risk management efforts are cohesive and effective.
For example, IT can provide technical expertise on the implementation and maintenance of AI systems, while legal can provide guidance on regulatory compliance and ethical considerations. By working together, organizations can identify and address potential risks more effectively.
Establishing Clear AI Risk Management Policies
Organizations should develop clear policies and guidelines for AI risk management. This will help to ensure that risk management efforts are consistent and aligned with the organization’s overall objectives.
Clear policies can include guidelines for data collection and storage, protocols for testing and validating AI systems, and procedures for addressing potential biases and errors. By establishing clear policies, organizations can promote transparency and accountability in their use of AI.
Overall, building a culture of AI risk management requires a commitment from all stakeholders to prioritize risk management and to work together to identify and mitigate potential risks. By doing so, organizations can harness the power of AI while minimizing its risks.
The Future of AI Risk Management
The field of AI risk management is evolving rapidly, and organizations need to stay up to date with the latest developments to effectively manage AI risks. Some areas for future consideration include:
Emerging Technologies and Their Impact on AI Risk
New technologies, such as quantum computing and blockchain, may impact AI risk management. Organizations need to monitor these trends and understand how they may impact their AI risk management strategies.
The Role of Government and Industry Regulations
Government and industry regulations will likely play an increasingly important role in AI risk management. Organizations need to stay up to date with the latest regulations and compliance requirements.
Preparing for the Evolving AI Risk Landscape
The AI risk landscape is constantly evolving, and organizations need to have a plan in place to adapt to these changes. This may involve developing robust risk management frameworks and regularly reviewing and updating policies and procedures.
Conclusion
Effective AI risk management is critical to the safe and responsible deployment of AI. By identifying and mitigating risks associated with AI, organizations can not only avoid harm to individuals, organizations, and society at large but also maximize the potential benefits of this transformative technology.