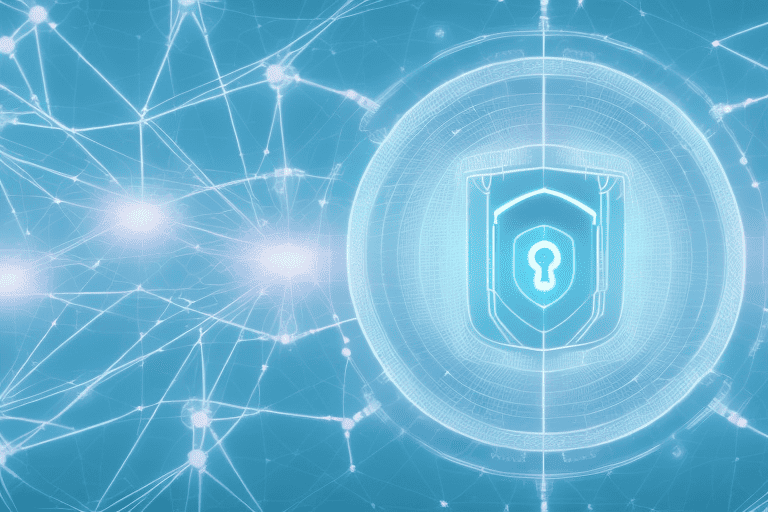
Exploring the Benefits of AI Network Security
Exploring the Benefits of AI Network Security
In today’s fast-paced digital world, network security has become more important than ever before. A single lapse in security can lead to catastrophic consequences, including financial loss, stolen data, and even legal liabilities. With the increase in sophisticated cyberattacks, traditional security solutions are no longer adequate and must be supplemented with innovative technologies such as Artificial Intelligence (AI).
Understanding AI Network Security
AI network security refers to the use of AI techniques to enhance traditional network security measures. It involves training computers to identify and block cyberattacks, including malware, phishing attempts, and other forms of cyber threats, by recognizing patterns in data that indicate malicious activity. AI network security systems use machine learning algorithms to analyze vast amounts of data from various sources, including devices, networks, and applications, in real-time. This allows them to detect and prevent threats that traditional security solutions may not be able to catch.
With the increasing number of cyber threats and attacks, AI network security has become crucial for organizations to protect their sensitive data and assets. The use of AI in network security has revolutionized the way organizations approach cybersecurity. AI network security systems provide a proactive approach to security, allowing organizations to detect and respond to threats before they cause any damage.
What is AI Network Security?
AI network security is a subfield of Artificial Intelligence and cybersecurity, focused on developing and deploying intelligent algorithms and models that can automate certain security-related tasks. These tasks include threat detection and prevention, incident response and remediation, and security operations optimization. By using AI techniques, network security systems can analyze data faster and more accurately than humans, enabling them to identify and respond to threats in real-time.
AI network security systems can also learn from experience, becoming smarter and more effective at detecting and mitigating threats over time. This is because they can analyze vast amounts of data and learn from patterns, making them more accurate and efficient in detecting and preventing cyber threats. Additionally, AI can automate certain security-related tasks, reducing the workload and associated costs for security teams.
How AI Enhances Traditional Network Security
AI enhances traditional network security in several ways. First, AI network security systems can identify and mitigate cyber threats in real-time, minimizing the risk of a breach. This is because AI systems can analyze vast amounts of data from multiple sources, allowing them to detect and prevent threats that traditional security solutions may not be able to catch.
Second, AI systems can learn from experience, becoming smarter and more effective at detecting and mitigating threats over time. This is because they can analyze vast amounts of data and learn from patterns, making them more accurate and efficient in detecting and preventing cyber threats.
Finally, AI can automate certain security-related tasks, reducing the workload and associated costs for security teams. This enables security teams to focus on more critical tasks, such as threat hunting and incident response, while AI takes care of routine tasks such as patching and updating systems.
Key Components of AI Network Security Systems
The key components of AI network security systems include:
- Big Data Analytics: AI network security systems aggregate and analyze vast amounts of data from various sources for insights and threat detection and response. By analyzing big data, AI systems can identify patterns and anomalies that may indicate a cyber threat.
- Machine Learning: AI network security systems use machine learning algorithms to recognize patterns and detect anomalies that indicate potential threats. By analyzing data and identifying patterns, AI systems can learn from experience and become more accurate and efficient in detecting and preventing cyber threats.
- Natural Language Processing (NLP): AI network security systems use NLP to identify and understand the context of written and spoken language in cybersecurity data. This allows AI systems to analyze and understand the content of emails, chat messages, and other forms of communication that may contain cyber threats.
- Behavioral Analytics: AI network security systems use behavioral analytics to identify abnormal behavior that may indicate a cyber threat. By analyzing user behavior and identifying anomalies, AI systems can detect and prevent insider threats and other forms of cyber attacks.
- Automation: AI network security systems automate certain processes, reducing the workload and associated costs for security teams. This includes tasks such as patching and updating systems, as well as incident response and remediation.
In conclusion, AI network security is a crucial component of modern cybersecurity. By using AI techniques such as machine learning, natural language processing, and behavioral analytics, organizations can enhance their traditional network security measures and protect their sensitive data and assets from cyber threats. With the increasing sophistication of cyber attacks, AI network security is becoming more important than ever for organizations to stay ahead of the curve and protect themselves from potential breaches.
Improved Threat Detection and Prevention
AI network security systems improve threat detection and prevention by providing real-time monitoring and analysis, identifying and mitigating zero-day vulnerabilities, and reducing false positives and false negatives.
Real-time Monitoring and Analysis
AI network security systems can analyze vast amounts of data in real-time, detecting and mitigating threats as they occur. By continuously monitoring systems for anomalies in network traffic and user behavior, AI systems can identify potential threats and respond quickly, preventing or minimizing the impact of a breach.
For example, let’s say that a company’s network security system is being monitored by an AI system. Suddenly, the system detects a large amount of data being transferred from one computer to another. The AI system immediately alerts the security team, who can investigate the issue and determine if it is a legitimate transfer or a potential data breach.
Identifying and Mitigating Zero-day Vulnerabilities
A zero-day vulnerability is a software security flaw that is unknown to the software vendor, making it difficult to defend against. AI network security systems can identify and mitigate these types of vulnerabilities by using behavioral analytics to detect unusual or abnormal behavior that may indicate a new type of attack.
For instance, let’s say that a company’s network security system is being monitored by an AI system. The AI system detects that a user is attempting to access a file that they have never accessed before. The AI system then analyzes the user’s behavior and determines that this is not typical behavior for the user. The AI system alerts the security team, who can investigate the issue and determine if it is a legitimate access attempt or a potential breach.
Reducing False Positives and False Negatives
Traditional security solutions have high rates of false positives and false negatives, which can lead to wasted resources and decreased confidence in the security team’s ability to detect and prevent threats. AI network security systems reduce false positives and false negatives by using machine learning algorithms to learn what constitutes normal network activity, and then detect and respond to anomalous behavior.
For example, let’s say that a company’s network security system is being monitored by an AI system. The AI system has been trained to identify normal network activity and behavior, and has learned what constitutes abnormal behavior. When the AI system detects abnormal behavior, it alerts the security team, who can investigate the issue and determine if it is a legitimate threat or a false positive.
In conclusion, AI network security systems are an important tool for improving threat detection and prevention. By providing real-time monitoring and analysis, identifying and mitigating zero-day vulnerabilities, and reducing false positives and false negatives, AI systems can help organizations stay one step ahead of potential cyber threats.
Enhanced Incident Response and Remediation
AI network security systems have revolutionized the way incident response and remediation is carried out in the cybersecurity industry. These systems have the ability to automate processes, accelerate remediation efforts, and continuously learn and adapt to new threats.
The need for enhanced incident response and remediation processes has become increasingly important due to the rise in cyber attacks. According to a report by Cybersecurity Ventures, cybercrime damages are expected to cost the world $6 trillion annually by 2021.
Automating Incident Response Processes
AI network security systems automate incident response processes, reducing the workload and associated costs for security teams. They can automatically detect and isolate affected systems or devices, and implement remediation strategies based on historical incident data. This means that security teams can focus on more complex tasks that require human intervention, while the AI system takes care of the routine tasks.
Moreover, AI systems can analyze vast amounts of data and identify patterns that would be difficult for humans to detect. This allows for faster and more accurate identification of security incidents, reducing the time it takes to respond and ultimately minimizing the impact of the breach.
Accelerating Remediation Efforts
AI network security systems accelerate remediation efforts by providing real-time analysis and threat detection. They can detect, contain, and mitigate threats quickly, preventing or minimizing the impact of a breach. In addition, AI systems can prioritize incidents based on their level of severity, allowing security teams to focus on the most critical incidents first.
Furthermore, AI systems can provide recommendations for remediation strategies based on historical data. This means that security teams can implement the most effective remediation strategies quickly and efficiently, reducing the time it takes to recover from an incident.
Continuous Learning and Adaptation
AI network security systems continuously learn and adapt, improving their ability to detect and prevent threats over time. They can analyze vast amounts of historical data to identify patterns and trends, and then use this information to enhance their threat detection and response capabilities.
Moreover, AI systems can learn from their mistakes. If a threat is not detected or remediated properly, the system can analyze the incident and identify where improvements can be made. This means that the system becomes more effective over time, providing better protection against cyber threats.
In conclusion, AI network security systems have transformed incident response and remediation processes in the cybersecurity industry. By automating processes, accelerating remediation efforts, and continuously learning and adapting, these systems provide faster and more effective protection against cyber threats. As cyber attacks become more sophisticated, AI systems will play an increasingly important role in protecting organizations from the devastating effects of cybercrime.
Cost Savings and Efficiency Gains
AI network security systems provide cost savings and efficiency gains by reducing manual labor and human error, streamlining security operations, and optimizing resource allocation.
With the increasing complexity and frequency of cyber attacks, organizations need to be proactive in their security measures. AI network security systems provide an effective solution by automating tasks that would otherwise require a significant amount of manual labor.
Reducing Manual Labor and Human Error
By automating certain security-related tasks, AI network security systems reduce the workload and associated costs for security teams. This allows security professionals to focus on more strategic tasks, such as threat analysis and response planning.
Moreover, AI network security systems reduce the risk of human error, which can lead to missed threats or false positives. This is particularly important in the context of complex security environments, where even small mistakes can have serious consequences.
Streamlining Security Operations
AI network security systems streamline security operations by automating incident response processes and supporting collaboration among security teams. This results in faster response times and more efficient use of resources.
For example, when an incident occurs, an AI system can automatically analyze the threat, classify it, and initiate the appropriate response. It can also provide relevant information to the security team, such as the source of the attack and any potential vulnerabilities that need to be addressed.
Optimizing Resource Allocation
AI network security systems optimize resource allocation by providing insights and recommendations regarding security-related investments. They can identify where resources are needed most, such as specific areas of vulnerability or increased threat activity.
Furthermore, AI systems can help organizations prioritize their security investments based on risk and potential impact. This allows organizations to allocate resources more effectively and efficiently, ultimately leading to better security outcomes.
Overall, AI network security systems are a valuable tool for organizations looking to improve their security posture while also reducing costs and increasing efficiency.
Challenges and Limitations of AI Network Security
Despite its potential benefits, AI network security faces several challenges and limitations. These include data privacy and security concerns, the potential for AI-driven cyberattacks, and the need to ensure AI system transparency and accountability.
As technology continues to advance, the amount of data that is collected and analyzed by AI network security systems is increasing. This raises concerns about data privacy and security, as well as the potential for data breaches or misuse. To address these concerns, companies must implement strict security measures and protocols to protect sensitive information from unauthorized access or theft.
Another challenge facing AI network security is the potential for AI-driven cyberattacks. Attackers can use AI algorithms to automate attacks, evade detection, and identify vulnerabilities more quickly. This can lead to more sophisticated and targeted attacks that are difficult to defend against.
Furthermore, ensuring AI system transparency and accountability can be a challenge. AI network security systems use complex algorithms and models that can be difficult to understand or explain. This can make it challenging to ensure transparency and accountability in their decision-making processes. To address this challenge, companies must prioritize transparency and accountability in their AI network security systems, and invest in technologies and tools that can help explain how decisions are made.
Data Privacy and Security Concerns
AI network security systems analyze large amounts of data, including sensitive information such as personal identifiers and financial data. This raises concerns about data privacy and security, as well as the potential for data breaches or misuse. To address these concerns, companies must implement strict security measures and protocols to protect sensitive information from unauthorized access or theft.
Companies must also ensure that they are complying with relevant data privacy regulations, such as the General Data Protection Regulation (GDPR) in the European Union. The GDPR requires companies to obtain explicit consent from individuals before collecting and processing their personal data, and to implement appropriate security measures to protect that data.
Potential for AI-driven Cyberattacks
The same AI algorithms that enhance network security can also be used to launch cyberattacks. Attackers can use AI to automate attacks, evade detection, and identify vulnerabilities more quickly. This can lead to more sophisticated and targeted attacks that are difficult to defend against.
To address this challenge, companies must invest in advanced AI-driven cybersecurity solutions that can detect and respond to AI-driven attacks. These solutions must be able to identify patterns and anomalies in network traffic, and respond quickly to mitigate any potential threats.
Ensuring AI System Transparency and Accountability
AI network security systems use complex algorithms and models that can be difficult to understand or explain. This can make it challenging to ensure transparency and accountability in their decision-making processes.
To address this challenge, companies must prioritize transparency and accountability in their AI network security systems. This includes investing in technologies and tools that can help explain how decisions are made, and ensuring that these systems are subject to regular audits and reviews.
Furthermore, companies must ensure that their AI network security systems are designed to be ethical and unbiased. This requires careful consideration of the data and algorithms used in these systems, and ongoing monitoring to ensure that they are not inadvertently perpetuating biases or discrimination.
Future Outlook and Industry Trends
The importance of AI in cybersecurity is likely to continue to grow in the coming years. Several emerging AI network security technologies are poised to make a significant impact on the field.
The Growing Importance of AI in Cybersecurity
The growing volume and complexity of cyber threats, combined with the increased use of connected devices, has made AI network security a critical component of cybersecurity. Companies and organizations that fail to adopt AI security solutions risk falling behind and becoming vulnerable to emerging threats.
Emerging AI Network Security Technologies
Several emerging AI network security technologies are expected to make significant contributions to the field, including:
- Deep Learning: Deep learning is a subfield of machine learning that uses advanced neural networks to learn from experience and make intelligent decisions.
- Explainable AI: Explainable AI is designed to make AI decision-making more transparent and understandable, helping to ensure accountability and build trust.
- AIIoT Security: AIIoT Security is a new approach to cybersecurity that combines AI and the Internet of Things (IoT) to secure connected devices and networks.
Preparing for an AI-driven Security Landscape
As AI network security continues to evolve, it is essential to remain vigilant and proactive in addressing emerging threats. This includes continually evaluating and refining existing security measures, adopting emerging technologies, and investing in ongoing training and education for security personnel.