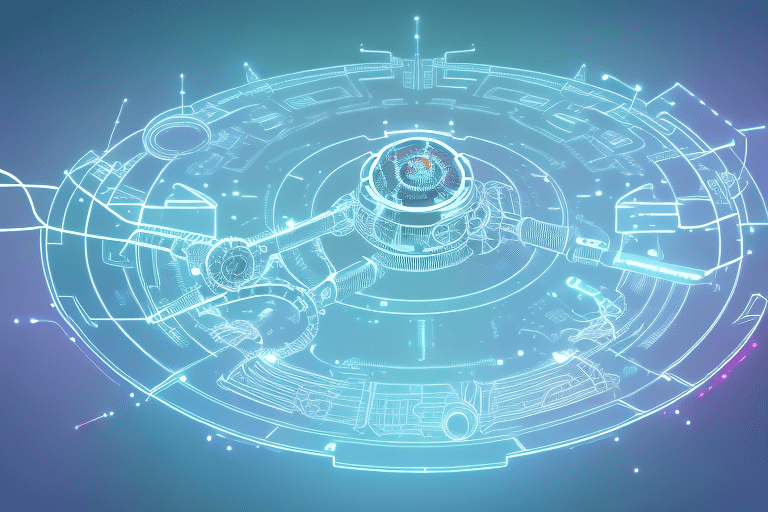
Overcoming AI Challenges in the Enterprise: A Comprehensive Guide
Overcoming AI Challenges in the Enterprise: A Comprehensive Guide
Artificial Intelligence (AI) holds the power to revolutionize the way organizations operate, innovate and grow. It is becoming increasingly essential for businesses to incorporate AI solutions to optimize processes, drive growth and gain competitive advantage. However, the implementation of AI in the enterprise comes with a range of challenges that need to be overcome to ensure its effective and ethical use. In this comprehensive guide, we take a detailed look at the main challenges of AI implementation in the enterprise and explore practical steps to overcome them.
Introduction to AI Challenges in the Enterprise
Artificial intelligence (AI) has been touted as the future of enterprise technology. However, implementing AI in a business environment is not without its challenges. While AI has the potential to revolutionize the way businesses operate, there are several factors that can hinder its implementation.
Data Management Issues
One of the most significant challenges that companies face when implementing AI is data management. AI algorithms require massive volumes of data to function correctly. However, many companies struggle to manage and organize the data that their AI systems require. This can result in delays and errors in the AI system’s output, which can have serious consequences for the business.
To overcome this challenge, companies need to invest in robust data management systems that can handle the volume and complexity of the data required for AI systems. This can involve hiring data management experts or investing in data management software to ensure that the data is organized and accessible to the AI system.
Training and Hardware Costs
Another significant challenge that businesses face when implementing AI is the high cost of training and hardware. AI systems require significant amounts of computing power and storage, which can be expensive for businesses on a tight budget. Additionally, training an AI system requires a significant investment of time and resources.
One way that businesses can overcome this challenge is by partnering with AI vendors who can provide pre-trained models and cloud-based AI services. This can significantly reduce the upfront costs of implementing AI, making it more accessible to businesses of all sizes.
Ethical Concerns
As AI becomes more prevalent in the enterprise, there are growing concerns about its ethical implications. For example, AI systems can perpetuate biases and discrimination if they are not designed and trained correctly. Additionally, there are concerns about the impact of AI on jobs and the economy.
To address these concerns, businesses need to be proactive in designing and implementing ethical AI systems. This can involve working with experts in AI ethics and ensuring that AI systems are designed to be transparent and accountable.
Integration and Interoperability Issues
Integrating AI systems into existing business processes can be a significant challenge. Many businesses have complex IT infrastructures with multiple systems that need to work together seamlessly. Additionally, different AI systems may not be compatible with each other, which can make it difficult to integrate them into a single system.
To overcome these challenges, businesses need to invest in robust integration and interoperability solutions. This can involve working with vendors who specialize in integrating AI systems into existing IT infrastructures and ensuring that different AI systems are designed to be compatible with each other.
AI Model Accountability
Finally, there is a growing concern about the accountability of AI models. As AI becomes more prevalent in the enterprise, it is essential to ensure that AI systems are transparent and accountable. This can involve implementing auditing and monitoring systems to track the performance of AI systems and ensure that they are functioning correctly.
To ensure AI model accountability, businesses need to work with experts in AI governance and ensure that their AI systems are designed to be transparent and accountable. This can involve implementing auditing and monitoring systems, developing clear guidelines for AI model development and deployment, and ensuring that AI systems are subject to regular review and evaluation.
Data Management and Quality Issues
For AI models to be effective, they need to be trained using high-quality data. However, many businesses have challenges with data management, with data scattered across different systems and in different formats.
Effective data management is crucial for businesses to derive meaningful insights from their data. When data is scattered across different systems and in different formats, it becomes difficult for businesses to use it effectively. This can lead to ineffective AI models that do not generate valuable insights.
One of the biggest challenges that businesses face is ensuring that their data is of high quality. Low-quality data can lead to inaccurate insights and predictions, which can have a significant impact on business operations. Therefore, it is essential for businesses to have a robust data-management system that incorporates data curation to ensure high-quality data.
Data privacy is another critical aspect of data management. With the increasing number of data breaches and cyber-attacks, businesses need to ensure that their data is secure and adheres to privacy regulations. This is especially important for businesses that deal with sensitive customer information such as healthcare and financial data. A data breach can not only lead to financial losses but also damage the reputation of the business.
Businesses need a robust data-management system that incorporates data privacy and data governance. Data governance ensures that the data is managed effectively, and all stakeholders are aware of the data policies and procedures. This helps to ensure that the data is used effectively and efficiently, and any potential risks are identified and mitigated.
Effective data management is crucial for businesses to derive meaningful insights from their data. Businesses need a robust data-management system that incorporates data curation, data privacy, and data governance to ensure high-quality, secured data.
Ensuring AI Ethics, Privacy, and Compliance
Artificial Intelligence (AI) is a rapidly developing technology that is transforming the way businesses operate. It has the potential to revolutionize industries, from healthcare to finance, and to improve people’s lives in many ways. However, as with any new technology, there are concerns about its impact on society and the risks it poses to privacy, bias, and discrimination.
AI ethics is becoming increasingly essential for businesses as they incorporate AI into their processes. Companies must ensure that their AI solutions are designed and implemented in an ethical and responsible way. This involves identifying potential ethical issues, such as bias and discrimination, and taking steps to mitigate them.
One of the most significant concerns around AI is its impact on privacy. AI systems have access to vast amounts of data, and there is a risk that this data could be misused or mishandled. To address this concern, companies must ensure that their AI solutions are designed with privacy in mind. This includes implementing robust data protection measures, such as encryption and access controls, to protect sensitive data.
Another important consideration for businesses is ensuring compliance with the latest regulations and standards. With the rise of regulatory compliance requirements around the world, the need to ensure AI compliance has become critical. Companies must prioritize ethical considerations in their AI solutions and ensure that they comply with the latest regulations and standards.
Ensuring AI compliance involves a range of activities, including risk assessments, compliance audits, and ongoing monitoring and reporting. Companies must also ensure that their AI solutions are transparent and explainable, so that stakeholders can understand how decisions are being made.
There are various frameworks and guidelines available to help companies ensure AI ethics, privacy, and compliance. For example, the European Union’s General Data Protection Regulation (GDPR) sets out strict requirements for the collection, processing, and storage of personal data. The IEEE Global Initiative on Ethics of Autonomous and Intelligent Systems has also developed a set of ethical guidelines for AI, which cover issues such as transparency, accountability, and fairness.
AI ethics, privacy, and compliance are critical considerations for businesses that are incorporating AI into their processes. Companies must prioritize ethical considerations in their AI solutions and ensure that they comply with the latest regulations and standards. By doing so, they can mitigate risks, build trust with stakeholders, and ensure that their AI solutions are designed and implemented in an ethical and responsible way.
Integration and Interoperability of AI Systems
Most organizations today understand the value of implementing AI systems to drive efficiencies and improve business outcomes. However, the integration and interoperability of these systems with other systems in the organization can be challenging.
One of the main challenges businesses face is the different architectures and technologies used by different systems. For example, one system may be on-premise, while another may be cloud-based. This can create data silos, making it difficult to access and share data across systems.
Another challenge is ensuring seamless workflow across systems. When AI systems are not integrated with other systems, it can lead to manual data entry, which can be time-consuming and prone to errors. This can reduce the efficiency of the development process and increase costs.
Businesses must address these integration and interoperability issues to fully leverage the benefits of AI. By breaking down data silos and ensuring seamless workflow across systems, businesses can access the data they need to make informed decisions and drive better business outcomes.
One way to address integration and interoperability issues is through the use of APIs. APIs can help connect different systems, allowing data to flow seamlessly between them. This can help businesses streamline their processes and reduce the time and effort required for manual data entry.
Another approach is to use middleware platforms. Middleware platforms can help bridge the gap between different systems by providing a common interface for data exchange. This can help businesses overcome the challenges of different architectures and technologies.
Ultimately, businesses must prioritize integration and interoperability when implementing AI systems. By doing so, they can fully leverage the benefits of AI and drive better business outcomes.
Scaling AI Solutions and Managing Costs
Scaling AI solutions can be a daunting task for businesses, especially those that lack expertise in AI development. However, the benefits of implementing AI solutions are numerous, ranging from increased efficiency to improved customer experience. Therefore, it is essential for businesses to overcome the challenges of scaling AI solutions.
One way to scale AI solutions is by leveraging the cloud. Cloud providers offer a range of services that can help businesses scale their AI solutions without incurring high costs. For instance, cloud providers offer scalable compute and storage resources, which can be easily provisioned and de-provisioned as needed. This allows businesses to pay only for the resources they use, which can help reduce costs.
Another way to scale AI solutions is by outsourcing parts of the development. Outsourcing can help businesses access expertise that they may not have in-house. For example, businesses can outsource the development of specific AI models or the management of their AI infrastructure. This can help reduce the cost of hiring and training new employees, as well as reduce the time it takes to develop and deploy AI solutions.
However, it is important for businesses to have a strategy for managing costs when scaling AI solutions. One way to do this is by setting clear goals and objectives for the AI solution. This can help businesses prioritize their investments and ensure that they are only investing in the areas that will provide the most value. Additionally, businesses should regularly review their AI infrastructure and usage to identify areas where costs can be reduced.
Another way to manage costs is by investing in automation. Automation can help reduce the time and cost of developing and deploying AI solutions. For example, businesses can use automated tools to build and train AI models, which can help reduce the time it takes to develop the models. Additionally, businesses can use automation to monitor and manage their AI infrastructure, which can help reduce the cost of maintenance.
Scaling AI solutions can be challenging, but it is essential for businesses to stay competitive. By leveraging the cloud, outsourcing parts of the development, and having a strategy for managing costs, businesses can scale their AI solutions in a cost-effective manner. Furthermore, investing in automation can help reduce the time and cost of developing and deploying AI solutions, making it easier for businesses to reap the benefits of AI.